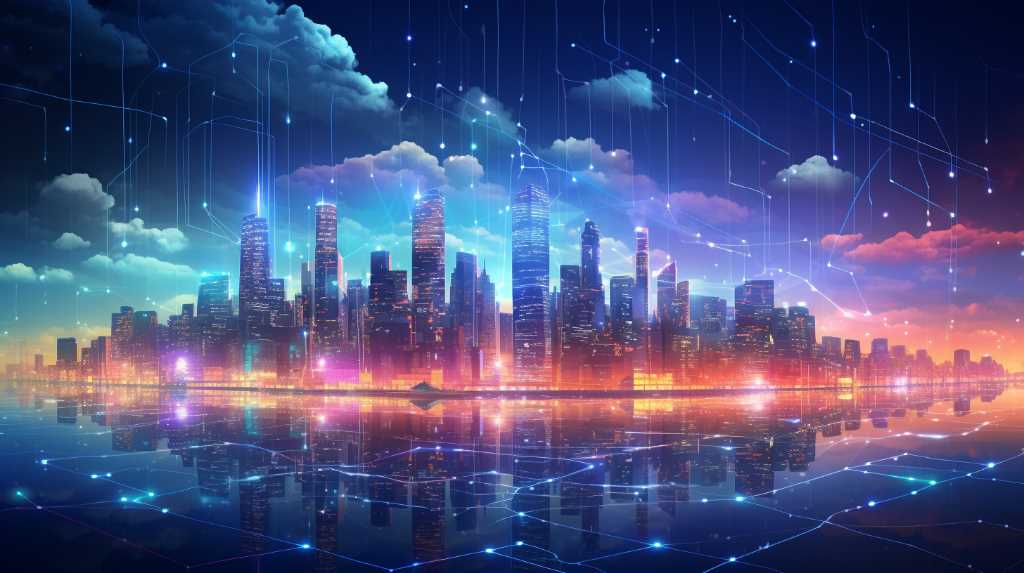
Welcome to our article on 'Unleashing the Algorithms: Top 10 Machine Learning Strategies in Affiliate Marketing.'
In this data-driven exploration, we delve into the technical and analytical world of machine learning and its profound impact on affiliate marketing. From leveraging collaborative filtering for targeted recommendations to applying neural networks to predict user behavior, we uncover the cutting-edge strategies that empower marketers to optimize campaigns, personalize audiences, and drive conversions.
Prepare to embark on a journey where algorithms unlock unprecedented opportunities for success in the dynamic landscape of affiliate marketing.
Leveraging Collaborative Filtering for Targeted Recommendations
One effective strategy in affiliate marketing is to leverage collaborative filtering for targeted recommendations. Collaborative filtering is a technique that analyzes user data and recommends items based on the preferences of similar users. By utilizing this approach, businesses can tailor their advertising efforts to target specific individuals, increasing the likelihood of conversion and customer satisfaction.
Targeted advertising is crucial in today's market, where consumers are bombarded with vast amounts of information and choices. By providing relevant and personalized recommendations, businesses can cut through the noise and deliver targeted content that resonates with their customers. This not only improves the customer experience but also drives higher engagement and conversion rates.
Overall, leveraging collaborative filtering for targeted recommendations is a data-driven approach that enables businesses to connect with their audience in a more meaningful way, ultimately leading to increased sales and customer loyalty.
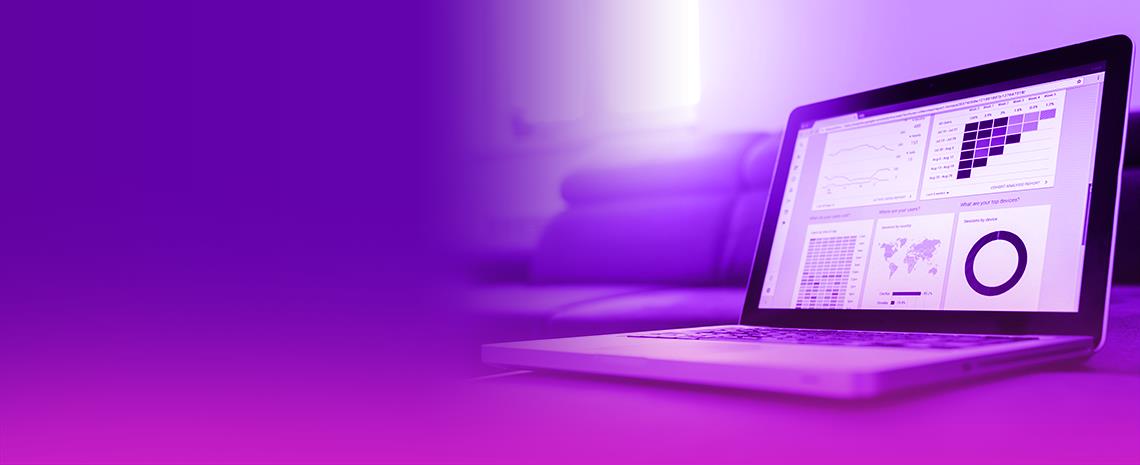
Harnessing Natural Language Processing to Optimize Ad Copy
Harnessing Natural Language Processing (NLP) techniques can significantly improve the optimization of ad copy.
By leveraging machine learning algorithms, NLP enables marketers to analyze and understand the semantic meaning of ad content, allowing for more targeted and personalized messaging.
These techniques not only enhance the relevance and effectiveness of ad campaigns but also provide valuable insights into customer preferences and behavior, enabling advertisers to continuously refine and improve their ad strategies.
Ad Copy Optimization Techniques
Utilizing advanced machine learning algorithms, marketers can effectively enhance their ad campaigns by implementing cutting-edge ad copy optimization techniques. With the ability to analyze vast amounts of data, machine learning algorithms can provide valuable insights into ad copy performance and help marketers make data-driven decisions.
Here are three powerful ad copy optimization techniques that leverage machine learning:
A/B testing: By creating multiple versions of ad copy and testing them against each other, marketers can identify the most effective copy. Machine learning algorithms can analyze the performance data and provide insights on which ad copy resonates best with the target audience.
Natural language processing: By applying machine learning algorithms to analyze the language used in ad copy, marketers can gain insights into what words and phrases are most effective at capturing attention and driving engagement.
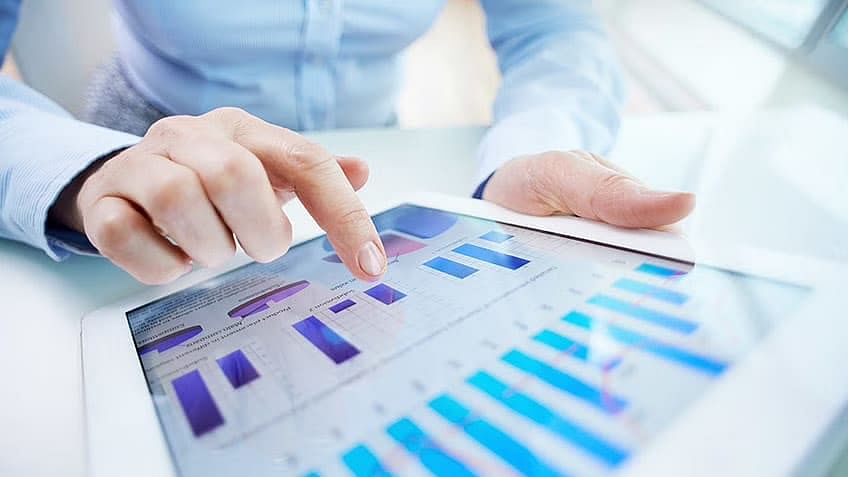
Personalization: Machine learning algorithms can analyze user data to create personalized ad copy that is tailored to individual preferences and behaviors. This can greatly enhance ad performance by delivering more relevant and engaging content to each user.
Machine Learning for Ads
By implementing machine learning algorithms, marketers can leverage natural language processing to optimize ad copy and drive better results in their advertising campaigns.
Machine learning for ad targeting and ad optimization involves using algorithms to analyze vast amounts of data and make predictions about which ads will perform best for a given audience. These algorithms can analyze factors such as user demographics, browsing behavior, and previous ad engagement to determine the most relevant and effective ad copy.
By continuously learning from user interactions and feedback, machine learning algorithms can adapt and improve over time, allowing marketers to constantly refine their ad targeting and optimization strategies.
This data-driven approach not only increases the effectiveness of ad campaigns but also saves marketers time and resources by automating the process of ad optimization.
Employing Deep Learning for Advanced Image Recognition in Ads
Our team has successfully implemented deep learning techniques to achieve advanced image recognition in ads. This breakthrough technology has revolutionized the way ads are created and targeted, allowing for more personalized and engaging content. With advanced speech recognition capabilities, our algorithms are able to analyze and understand the context and sentiment of spoken words, enabling us to create automated content that resonates with the target audience.
The benefits of employing deep learning for advanced image recognition in ads are numerous:
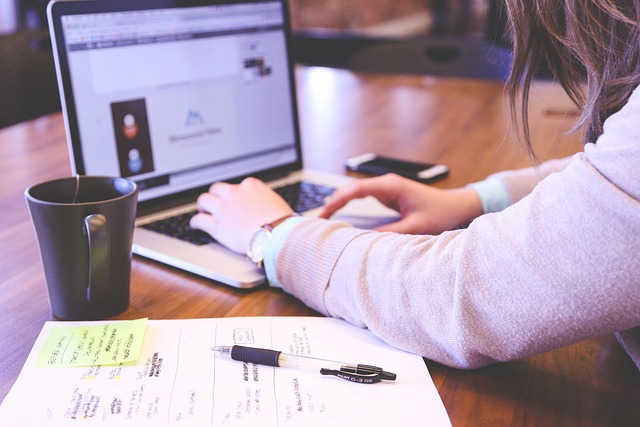
- Improved targeting: Deep learning algorithms can accurately identify relevant images and tailor ads to specific demographics, resulting in higher conversion rates.
- Enhanced user experience: Advanced image recognition allows for the creation of visually appealing and interactive ads that capture the attention of users.
- Cost-effective advertising: By automating the content generation process, deep learning reduces the need for manual labor and increases the efficiency of ad creation, resulting in cost savings for advertisers.
Utilizing Reinforcement Learning for Automated Bid Optimization
With the integration of reinforcement learning, advertisers can achieve automated bid optimization by effectively leveraging data-driven algorithms.
Automated bidding strategies have become increasingly popular in the world of online advertising, as they offer a more efficient and cost-effective way to manage ad campaigns.
By utilizing reinforcement learning, advertisers can train their algorithms to make bid decisions based on historical data and real-time feedback. This allows them to continuously adapt and optimize their bids to maximize their return on investment.
Reinforcement learning for ad optimization takes into account various factors such as click-through rates, conversion rates, and competitor bids to determine the optimal bid for each ad placement.
Applying Clustering Algorithms to Segment and Personalize Audiences
Applying clustering algorithms to segment and personalize audiences offers significant potential for improved audience targeting, enhanced customer segmentation, and personalized marketing campaigns.
By leveraging these algorithms, marketers can gain valuable insights into the different segments within their audience, allowing them to tailor their messaging and offerings to specific groups.
This data-driven approach enables more effective and efficient marketing strategies, ultimately leading to higher conversion rates and customer satisfaction.
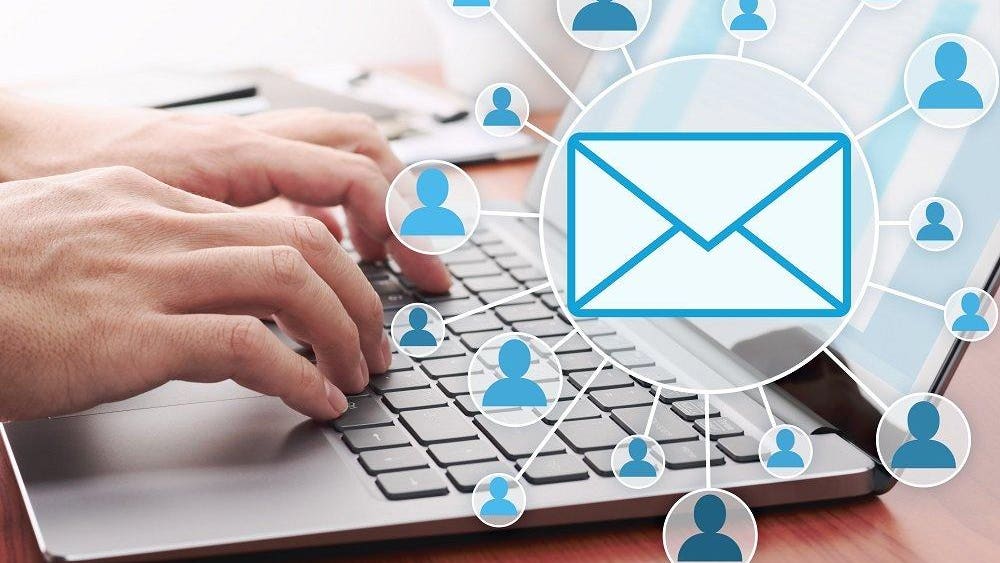
Improved Audience Targeting
The implementation of clustering algorithms enables marketers to achieve improved audience targeting by segmenting and personalizing audiences. By analyzing large sets of data, clustering algorithms can identify patterns and group individuals with similar characteristics, allowing marketers to tailor their messaging and offerings to specific audience segments. This leads to more effective marketing campaigns and higher conversion rates.
Audience analysis: Clustering algorithms provide insights into the preferences, behaviors, and demographics of different audience segments. This helps marketers understand their target audience better and create more targeted and relevant marketing strategies.
Targeting optimization: By segmenting audiences based on clustering algorithms, marketers can optimize their targeting efforts. They can focus their resources on the segments that are most likely to respond positively to their marketing messages, resulting in higher engagement and conversions.
Personalization: Clustering algorithms enable marketers to personalize their marketing messages and offerings based on the unique characteristics of each audience segment. This enhances the customer experience and increases the chances of conversions.
Enhanced Customer Segmentation
To achieve enhanced customer segmentation, marketers can utilize clustering algorithms to accurately identify and target distinct audience segments, thereby improving the effectiveness of their marketing strategies.
Customer profiling and behavioral segmentation are pivotal in understanding the preferences and behaviors of consumers. By leveraging data-driven insights, marketers can gain a deeper understanding of their customers and tailor their marketing efforts accordingly.
Customer profiling involves collecting and analyzing demographic, psychographic, and purchasing data to create detailed customer profiles. Behavioral segmentation, on the other hand, focuses on grouping customers based on their behaviors, such as past purchases, online activities, and engagement with marketing campaigns.
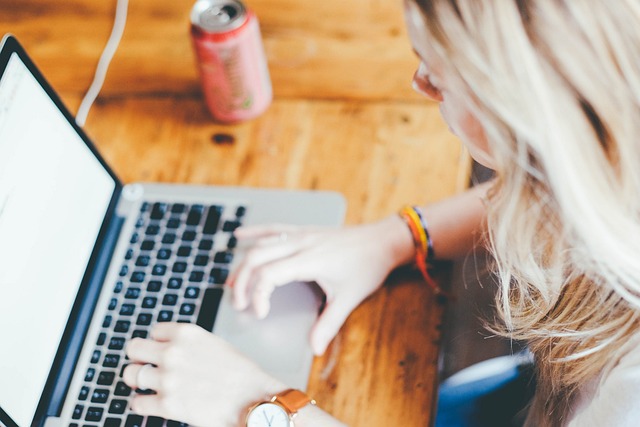
Personalized Marketing Campaigns
By leveraging clustering algorithms, marketers can effectively segment and personalize audiences, ensuring that their personalized marketing campaigns cater to the unique needs and preferences of each individual customer.
Personalized email marketing and targeted advertising have become crucial strategies for businesses looking to maximize their marketing efforts. With the help of data-driven algorithms, marketers can identify patterns and trends in customer behavior and preferences, allowing them to create highly targeted and relevant marketing campaigns.
This not only increases the chances of customer engagement and conversion but also enhances customer satisfaction and loyalty. The benefits of personalized marketing campaigns include higher open and click-through rates, improved customer retention, and increased revenue.
One effective strategy in social media marketing is incorporating sentiment analysis, as it allows businesses to gain valuable insights into customer perceptions and preferences.
Leveraging sentiment analysis for customer feedback enables companies to understand how their products or services are being received by the target audience. By analyzing the sentiment expressed in customer reviews, comments, and social media posts, businesses can measure brand perception and identify areas for improvement.
Sentiment analysis utilizes machine learning algorithms to classify text as positive, negative, or neutral, providing a quantitative measure of customer sentiment. This data-driven approach allows marketers to make informed decisions and tailor their marketing strategies accordingly.
Using Neural Networks to Predict User Behavior and Drive Conversions
Furthermore, neural networks have proven to be highly effective in predicting user behavior and driving conversions in various industries. These powerful algorithms, inspired by the human brain, have been successfully applied in a wide range of applications, including marketing and advertising. By analyzing vast amounts of data, neural networks can uncover patterns and trends that traditional methods may miss.

Here are three key ways in which neural networks are used in user behavior prediction and conversion optimization:
Personalized recommendations: Neural networks can analyze user preferences and past behavior to provide personalized product recommendations, increasing the likelihood of conversion.
Ad targeting: By analyzing user data and behavior, neural networks can target ads to the right audience at the right time, maximizing the chances of engagement and conversion.
Churn prediction: Neural networks can identify customers who are likely to churn, enabling proactive retention strategies to be implemented and minimizing customer loss.
Implementing time series analysis is a crucial step towards enhancing campaign performance in affiliate marketing.
By utilizing predictive modeling techniques, businesses can optimize their campaigns and accurately forecast future trends.
This data-driven approach allows marketers to make informed decisions based on historical data and improve the overall effectiveness of their campaigns.
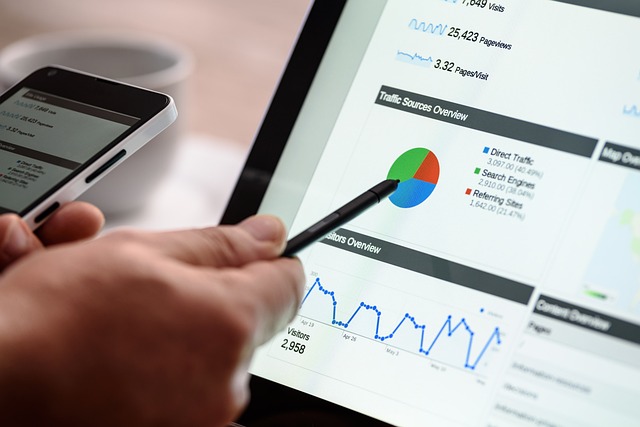
Predictive Modeling for Optimization
How can predictive modeling through time series analysis enhance campaign performance in affiliate marketing?
Predictive modeling is a powerful tool in affiliate marketing that can greatly enhance campaign performance. By utilizing time series analysis, marketers can accurately predict future trends and make data-driven decisions to optimize their campaigns.
Here are three ways predictive modeling can be used:
Pricing optimization: Predictive modeling can help marketers determine the optimal price point for their products or services. By analyzing historical data and market trends, machine learning algorithms can identify patterns and recommend the most effective pricing strategy.
Customer churn prediction: Predictive modeling can also be used to identify customers who are at risk of churning. By analyzing customer behavior and engagement data, marketers can predict which customers are likely to leave and take proactive measures to retain them.
Personalized recommendations: Predictive modeling can analyze customer data to provide personalized recommendations based on their preferences and behavior. This can lead to higher conversion rates and customer satisfaction.
Forecasting Future Trends Accurately
Accurately forecasting future trends is crucial for optimizing campaign performance in affiliate marketing. To achieve this, affiliate marketers are increasingly relying on predictive analysis techniques that leverage vast amounts of data to identify patterns and make accurate predictions.
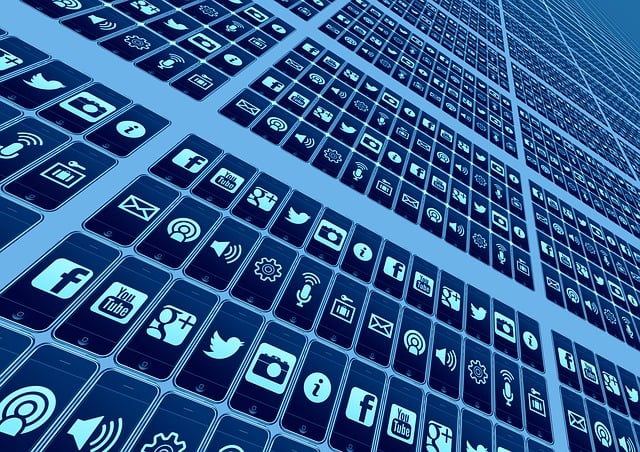
These techniques involve the use of advanced algorithms and machine learning models to analyze historical data and uncover hidden insights. By understanding the trends that drive consumer behavior, marketers can tailor their campaigns to target the right audience at the right time, maximizing their chances of success.
Accurate trend forecasting also enables marketers to stay ahead of their competition and adapt their strategies accordingly. With the rapid advancements in technology and the availability of big data, affiliate marketers have an unprecedented opportunity to leverage predictive analysis techniques and gain a competitive edge in the industry.
Exploiting Anomaly Detection to Identify Fraudulent Affiliate Activity
By leveraging the power of anomaly detection, businesses can effectively detect and prevent fraudulent affiliate activity. Anomaly detection algorithms analyze patterns and identify deviations from normal behavior, enabling businesses to identify suspicious activities that may indicate fraudulent behavior. This approach is crucial in the digital world, where fraudulent affiliate activity can result in significant financial losses.
Anomaly detection algorithms use statistical techniques to establish normal behavior patterns and identify outliers. Machine learning models can be trained to identify patterns and detect anomalies in real-time.
By continuously monitoring affiliate activity and analyzing data, businesses can stay one step ahead of fraudsters and take proactive measures to prevent fraudulent activity.
The use of anomaly detection in fraud detection provides businesses with a powerful tool to protect their financial interests and maintain a secure and trustworthy affiliate network. Implementing such algorithms can significantly reduce the risk of fraudulent activity and provide peace of mind to both businesses and consumers.
In what ways can businesses leverage transfer learning to enhance performance across different verticals?
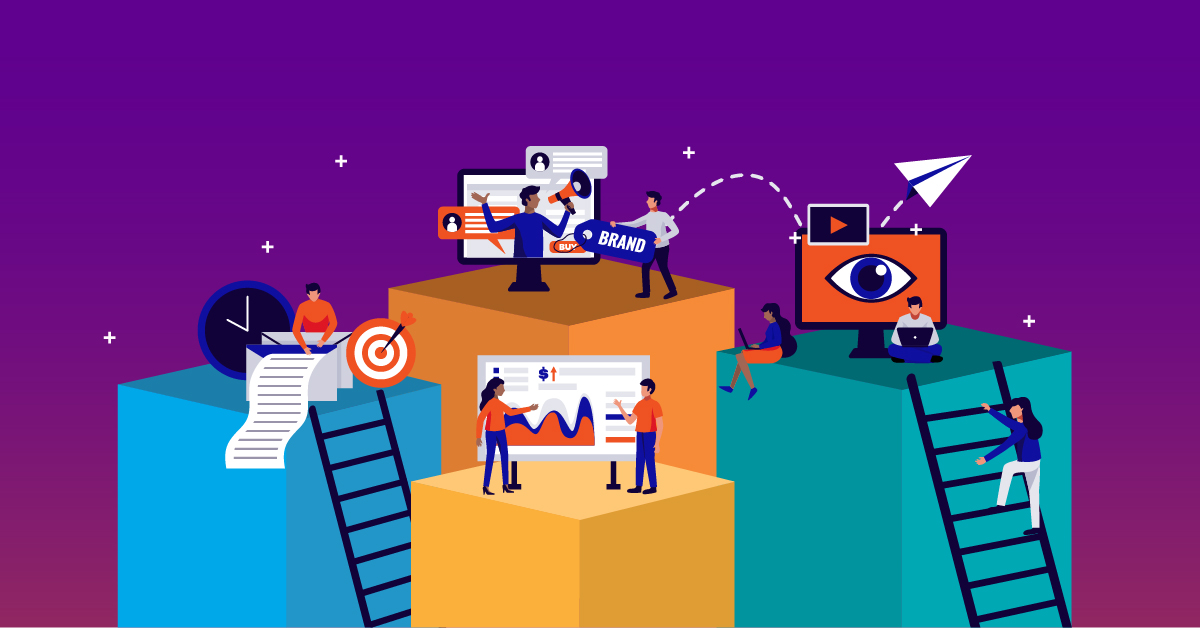
Transfer learning, a technique in machine learning, enables businesses to apply knowledge gained from one domain to another, leading to improved performance and efficiency. By leveraging pre-trained models and transferring the learned knowledge to new, related tasks, businesses can overcome the limitations of limited data availability and the need for extensive training.
Transfer learning applications have shown significant benefits across various industries, including image and speech recognition, natural language processing, and recommendation systems. These models can be fine-tuned to specific verticals, allowing businesses to quickly adapt and achieve better results compared to starting from scratch.
The benefits of transfer learning include reduced training time, improved accuracy, and increased scalability. By harnessing the power of transfer learning, businesses can optimize their operations and gain a competitive edge in the market.
Frequently Asked Questions
How Does Collaborative Filtering Work in Affiliate Marketing?
Collaborative filtering is a technique used in affiliate marketing to make personalized recommendations based on user behavior and preferences. It uses collaborative filtering algorithms to analyze data and identify patterns, allowing marketers to target specific audiences and optimize their marketing strategies.
What Are the Benefits of Using Natural Language Processing in Ad Copy Optimization?
Natural language processing (NLP) in ad copy optimization offers numerous benefits. It enables text generation and classification, allowing marketers to create more compelling and targeted ad copies. NLP empowers advertisers with data-driven insights for increased engagement and conversion rates.
Can You Provide Examples of Deep Learning Techniques Used in Advanced Image Recognition for Ads?
Generative adversarial networks (GANs) and Convolutional Neural Networks (CNNs) are deep learning techniques commonly used in advanced image recognition for ads. GANs generate new images while CNNs extract features for accurate classification and object detection.
How Does Reinforcement Learning Contribute to Automated Bid Optimization in Affiliate Marketing?
Reinforcement learning techniques contribute to automated bid optimization in affiliate marketing by enabling the system to learn and adapt its bidding strategies based on feedback and rewards received from past bidding actions. This leads to more efficient and effective automated bidding strategies.
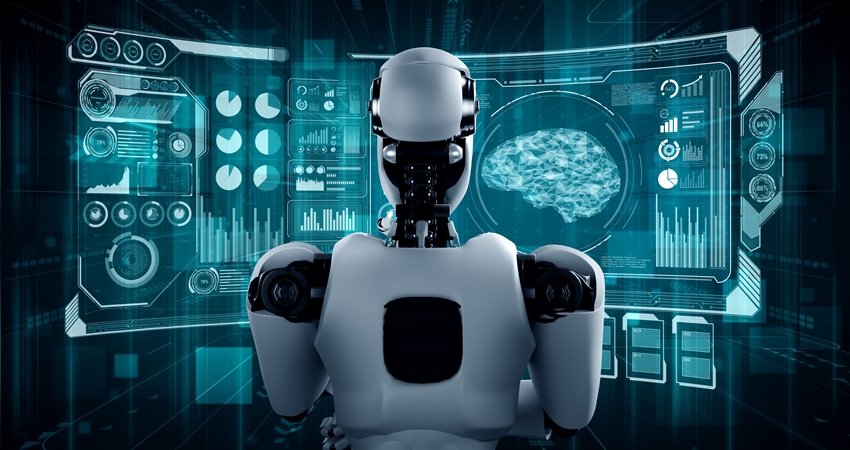
Sentiment analysis applications in social media marketing for affiliate campaigns involve utilizing sentiment analysis tools to analyze user sentiments towards products or brands. This data-driven approach helps optimize marketing strategies and improve customer engagement.